Ph.D. Student's Research Can Reduce Wind Turbine Costs by $15M Annually
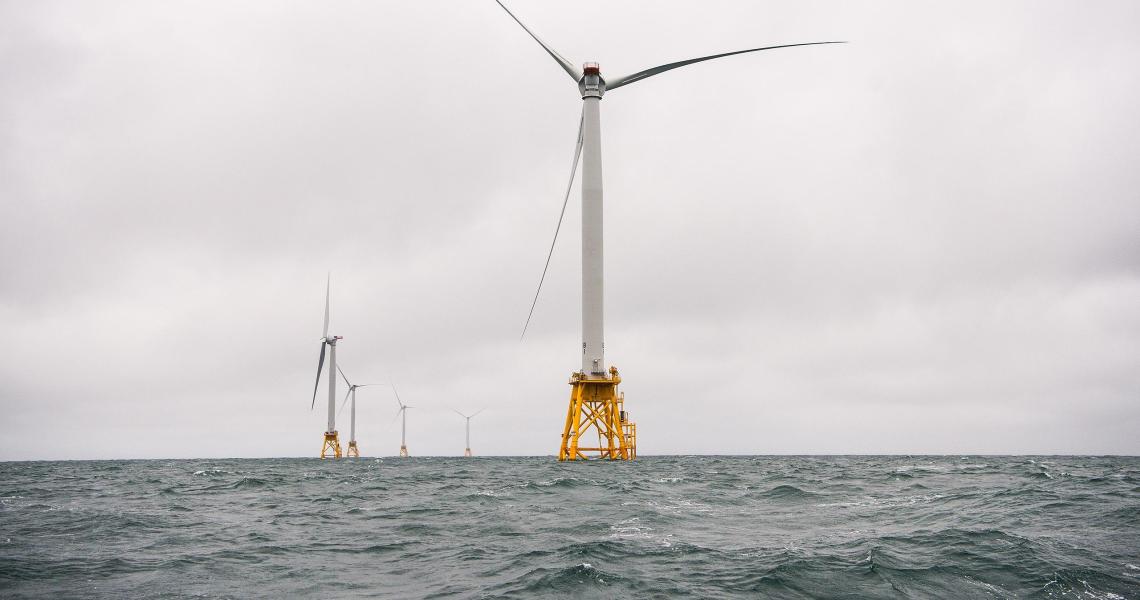
NJIT student Akhyurna Swain has a plan to save $15 million per year in the maintenance and operational costs of New Jersey's offshore wind turbines.
Swain, studying for her Ph.D. in electrical engineering, determined that non-intrusive monitoring technology controlled by magnets can be paired with machine learning software to realize the impressive cost savings for an 1,100-megawatt project which will begin construction in 2024.
Windmill technology began in the first millennium of the common era, but modern high-tech turbines reach a new plane of productivity. They'll be located 15 miles off Cape May and will provide energy for up to a half-million homes. Swain said that in monitoring the conversion of wind into electricity, most systems today use current-sensing apparatus or electrical clamps and coils, which are considered intrusive. She explained how the cost reductions and time savings could be obtained with her unobtrusive magnetic method to detect improper vibrations.
"It was observed that the components of the wind turbine-generator drive train are coupled with the common magnetic flux emanating from the wind generator. Thus, the magnetic flux distribution could be measured to detect faults on multiple components of the drive trains," Swain wrote in her research proposal.
"Multiple magnetoresistive sensors will be mounted on the external surface [of wind generators] to capture the magnetic flux leakage signals," which emanate from the generators, turbines and subsidiary components. "The vibrations induced by mechanical faults from the gearbox, bearings, etc., in the wind turbine drive train will modulate the magnetic field of the generator due to the electromechanical coupling between the drivetrain and the generator," she continued. "The air-gap torque of the generator links the electromagnetic and mechanical characteristics of the wind turbine drivetrain."
The results would be evaluated with artificial intelligence to determine what broke and where it happened. "This fault data acquired from the magnetic sensors is fed to a semi-supervised machine learning classifier to extract higher level representation of input fault features from the faulty magnetic field data signature for accurate fault classification," she stated.
"It's something very close to my heart. Even where I stayed [in India], it's the capital of a state and we had intermittent power. … Renewable energy is the need of the hour," Swain noted. Her methods would not impact avian or aquatic life, she added.
The doctoral research at NJIT is funded by New Jersey's Wind Institute Fellowship, which also funds research at Montclair State University, Rutgers University and Rowan University. Her work formally begins this fall. It's mostly in a laboratory for now, but Swain said she would love to climb one of the 900-foot turbine towers someday. They're being built by a company called Ørsted along with local energy utility PSEG, under the project name Ocean Wind 1. System testing is planned for summer 2023.
Swain studies under Associate Professor Philip Pong and alongside fellow students Sindhu Sai Sree Parimi and Salma Yadri Alami.