Computational Advance Will Help Researchers Model Climate With Higher Fidelity
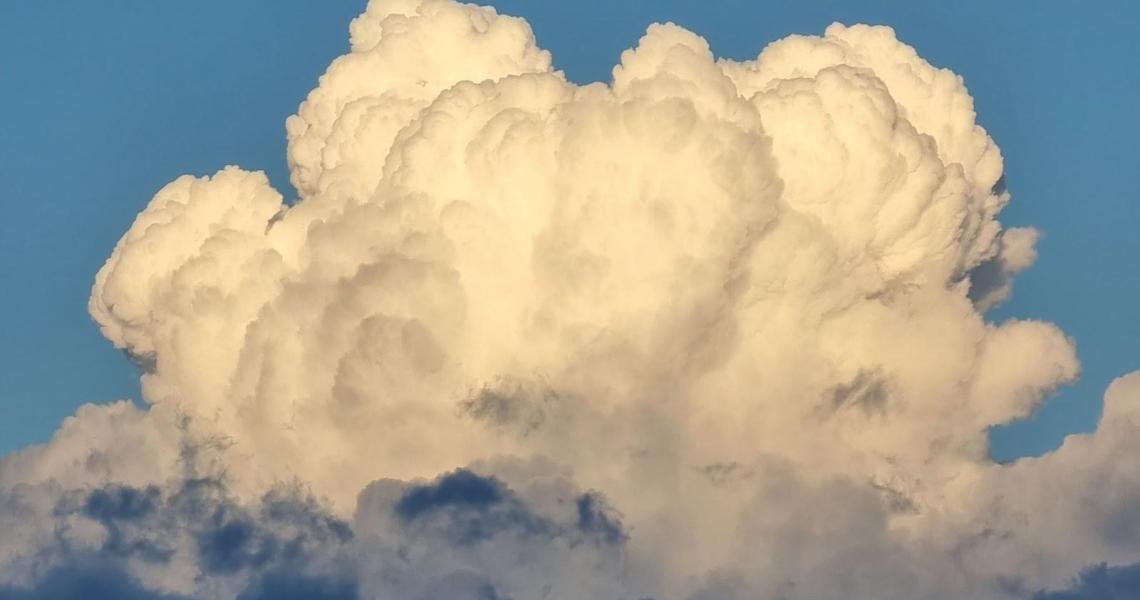
Increased computational power has dramatically improved climate modeling over the past several years, allowing scientists to better forecast long term climate evolution. Despite computers becoming faster and more efficient, cloud modeling was still a hazy prospect until a recent algorithmic improvement.
In a study published by Journal of Advances in Modeling Earth Systems, Ph.D. student Yassine Tissaoui from NJIT and collaborators, proposed a solution that balances computational resources with dynamic and precise cloud modeling — a notoriously difficult, yet crucial, aspect of climate modeling.
Clouds have a significant and complex impact on the Earth's energy balance and climate systems, acting as both a cooling and warming agent for the Earth’s surface and atmosphere.
Simulating the formation, evolution and behavior of clouds in climate models helps scientists understand the various processes that influence cloud formation, such as moisture availability, temperature and atmospheric dynamics.
Cloud modeling covers a wide range of space and time scales, making it challenging to capture their behavior accurately in climate models. Small-scale processes within clouds, such as cloud microphysics and turbulence, require complex calculations to approximate their effects on a larger scale. These calculations, referred to as parameterizations, are continually refined and updated based on observations and cloud modeling studies to improve the fidelity of climate models.
Previous modeling, however, is constrained by grid regularity — the boundaries on which the equations are solved.
As more powerful computers become available, these models are being run at higher resolutions, decreasing the distance between neighboring grid points that allow finer features to be resolved.
“We could theoretically use any number of grid points to subdivide the atmosphere and solve the equations at hand, but in practice we cannot afford it even on the largest supercomputers,” said Simone Marras, co-author of the paper and assistant professor in the department of mechanical and industrial engineering at New Jersey Institute of Technology.
Grid refinement becomes a necessary tool to use to keep computational costs down. However, legacy models are unable to handle rain when the grid’s regularity is “broken” by the dynamic redistribution of the grid points. The team’s work on computational algorithms solves this problem.
Caption: A 3D mature storm two hours into a simulation with the new implementation. The clouds are colored in gray, the semi-transparent blue shading represents the presence of rain, and the light and dark blue shadings represent temperature, showing where the air is cold due to evaporating rain. A sample of the 3D unstructured grid used for the simulation is shown in the background. Credit: Tissaoui et al. [2023]
Models will now be able to account for dynamic grid points, allowing for high-resolution modeling in some scenarios and lower-resolution refinement when appropriate without sacrificing overall fidelity. While this approach is well known in industrial applications of computational fluid dynamics, it is this work that leverages its advantages towards improved climate models.
The paper and its findings were also featured on Eos, the science news magazine published by the American Geophysical Union, as an editor highlight.
Citation: Tissaoui, Y., Marras, S., Quaini, A., de Brangaca Alves, F. A. V., & Giraldo, F. X. (2023). A non-column based, fully unstructured implementation of Kessler’s microphysics with warm rain using continuous and discontinuous spectral elements. Journal of Advances in Modeling Earth Systems, 15, e2022MS003283. https://doi.org/10.1029/2022MS003283