NJIT Experts Presenting AI Answers to Real-World Problems at NYC Forum
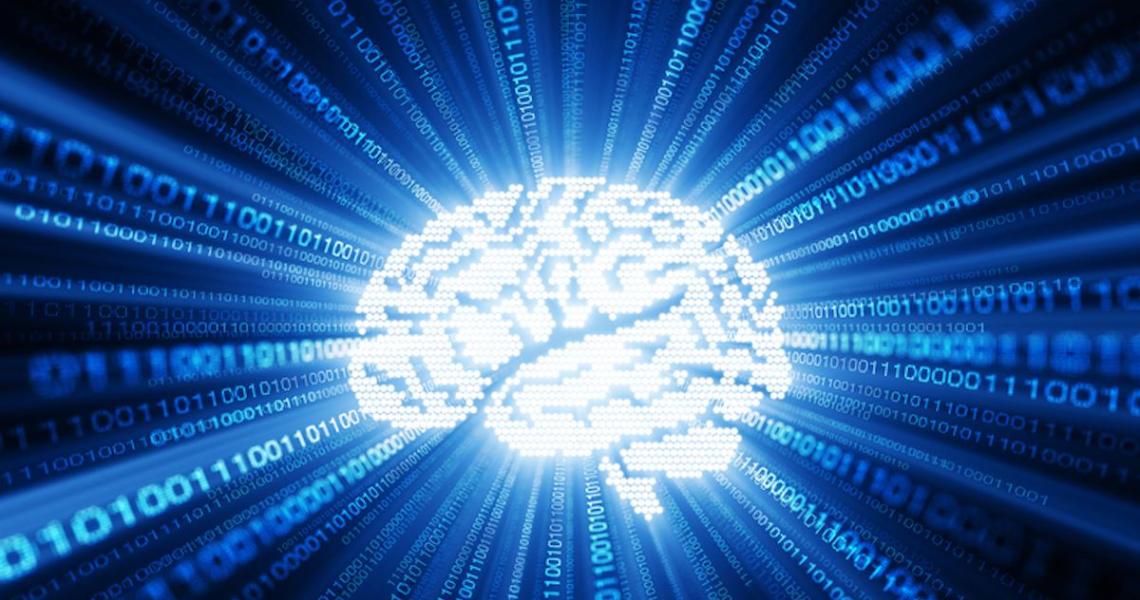
Experts in artificial intelligence from the Ying Wu College of Computing will highlight how their work solves real-world problems at a prestigious meeting in New York next week.
The professors — Chaoran Cheng, Jing Li, Zhi Wei and Pan Xu — will share their session stages with researchers from IBM, Facebook, Yahoo and other prominent organizations for audiences at the 34th annual conference of the Association for the Advancement of Artificial Intelligence.
"Today, artificial intelligence is great at learning patterns, such as making movie recommendations and identifying friends in our photographs. These technologies also enable futuristic self-driving cars and robotic helpers. These examples are just the beginning," said David Bader, director of NJIT's Institute for Data Science.
Looking forward a few years, the technology's predictive nature will become more reliable and better protect our privacy, Bader said.
At the conference, Wei and Cheng are jointly presenting on using machine learning software to compare genetic mutations in newborns with documentation of mutations in medical literature. In some cases, mutations are so rare that they are not yet cataloged or annotated in current databases. Geneticists need to manually search relevant literature to understand the mutations and then develop personalized medicine. The proposed algorithm would help to recognize relevant mutations and substantially expedite the search.
Wei is also using artificial intelligence to help researchers in China who are testing air quality monitoring devices controlled by mobile phones. The devices aren't very accurate, but when their data is taken in aggregate it can be corrected by artificial intelligence and made to show important trends. That's cheaper and more accessible than using a lesser amount of more expensive monitoring tools. The data is then presented to authorities who could suggest laws and policies to improve air quality.
"The model will learn from the past and try to make some predictions ... just like a student who learns from practice," Wei explained.
Li's topic is about how to improve allocation of server resources when a program has high data streaming requirements, such as those that need real-time speed or that are widely distributed. Her method uses a type of artificial intelligence called deep reinforcement learning, which is good for solving problems that involve extreme scalability challenges.
"To maximize system throughput, the resource allocation strategy that partitions the computation tasks of a stream-processing graph onto computing devices must simultaneously balance workload distribution and minimize communication," she stated.
A graph in this context works like a map indicating to the software where and how to arrange its resources in the most efficient way. Li and her colleagues use a graph neural network as the mapping function and reinforcement learning to optimize the function. She said this is the first time where an artificial intelligence component has achieved success in this context.
Xu's application of artificial intelligence is designed to understand discrimination in ride-booking services such as Lyft and Uber. Most people think of discrimination in terms of drivers declining passengers based on race or ethnicity. However, Xu said the most common example is when passengers of any background are declined because their requested trips are too short or out-of-the-way to be worthwhile for drivers looking to maximize profit. That problem always existed in traditional taxicabs, but now it's easier to study due to the availability of data.
Lyft and Uber drivers are prevented by company policies from canceling trips due to the length, so instead they delay their arrival in hopes that passengers will do the canceling and hire someone else, Xu explained. Conventional discrimination, of course, is also still a problem — data analyzed by artificial intelligence shows that African-American riders have longer wait times, he said. Software helps determine all of this because the allocation of rides is one big real-time decision-making puzzle, Xu noted.