Ioannis Koutis Receives ICCAD Best Paper Award
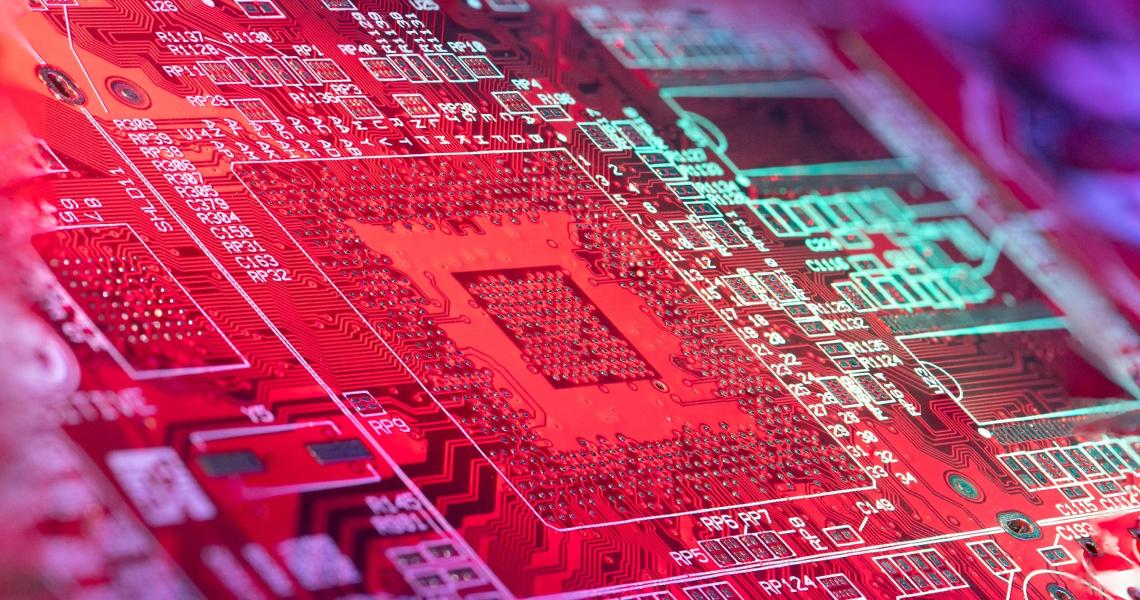
Ioannis Koutis, associate professor in the Department of Computer Science, was the recipient of the William J. McCalla Best Paper Award (back end) at the 2022 ACM/IEEE International Conference on Computer-Aided Design (ICCAD), held in November in San Diego, along with collaborators Ismail Bustany from Advanced Micro Devices (AMD), and Andrew Kahng, Bodhisatta Pramanik, and Zhiang Wang from the University of California San Diego (UCSD).
ICCAD is the premier forum to explore new challenges, present leading-edge innovative solutions, and identify emerging technologies in the electronic design automation research areas. The conference covers the full range of Computer Aided Design topics – from device and circuit-level up through system-level, as well as post-CMOS design, and is a top conference in the area of Electronic Design Automation (EDA), as reflected in csrankings.org, a widely accepted ranking reference for Computer Science.
Koutis is an expert in numerical algorithms for networks. These algorithms are capable of handling very large networks with practical applications in Graph Analytics, Computational Biology, and Electronic Design Automation.
The award-winning paper presented by Koutis and his collaborators, selected from over 125 accepted papers, explores hypergraph partitioning, a key problem in EDA. Hypergraphs are abstract representations of electronic circuits, and their partitioning allows to split a circuit into parts of roughly equal size while minimizing the connections among parts. This leads to a “divide-and-conquer” approach to more efficient designs, requiring less communication between physically distant parts of the circuits, in turn generating an overall positive effect on speed and other measures of performance. Hypergraph partitioning is so critical, that even relatively small improvements in it may lead to economies of scale.
Koutis said, “Our work can ultimately be used to improve EDA pipelines. However, this is only the first step in a research program that aims to explore the full power of advanced numerical network algorithms and machine learning methods in the context of EDA.”