Engineering Professor Helps Invent New Methods of Data Management
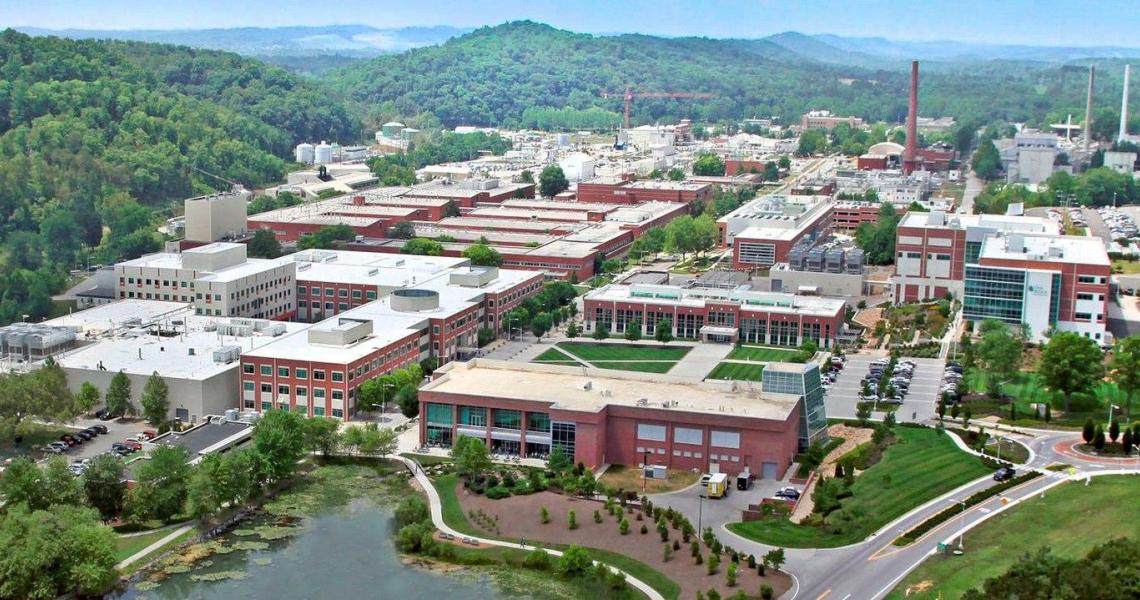
Reflect for a moment on how you'd manage your terabyte-scale hard drive if the data were literally one million times bigger.
Then you'd be working with exabytes, which is the reality for supercomputer users at federal laboratories across the country, one of which is relying on Assistant Professor Qing Liu to mitigate their information storage problems by studying new methods of data reduction.
Liu, along with Oak Ridge National Laboratory Distinguished Scientist Scott Klasky, is aiming to see what happens when data from research such as fusion experiments and climate change models is compressed at rates far beyond your average .zip file. Current compression schemes handle ratios such as 10:1 or 100:1, but the people in Liu's world want to know what happens with ratios in the thousands.
The more you squeeze a file, the more likely it is to lose important information, Liu explained. He needs to know at what point the loss makes the file compression not worthwhile. Losing a few bits in your Photoshop image might not be noticeable, but the stakes are higher when it comes to supercomputer applications such as measuring nuclear fallout or predicting several feet of rising tides.
“Data is our precious resource, and we need to make sure that we don’t make incorrect conclusions just to save storage. We need to make sure that the analysis will be accurate, which makes scientific data compression much more challenging compared to video compression,” Klasky noted.
"The novelty here is that we also evaluate how the outcomes of data analysis will change, in addition to compression ratio, speed and the standard error metrics," Liu added. Getting efficient data reduction from supercomputers also reduces the time-to-knowledge for scientific discovery, he added.
Liu is well-positioned to find the answers as a faculty member in NJIT's Department of Electrical and Computer Engineering and at the Oak Ridge Computer Science and Mathematics Division. He is currently the principal investigator on three related grants totalling more than $900,000, with two from the Department of Energy and one from the National Science Foundation.
The funds support graduate students such as Zhenbo Qiao, who now works at Amazon, and Jinzhen Wang, who's joining Los Alamos National Laboratory as a summer intern. Graduate students Weiming He and Zhenlu Qin are also participating, along with additional collaborators including the laboratory's Ben Whitney, Qian Gong, Lipeng Wan and Ruonan Wang, plus Xin Liang from Missouri University of Science and Technology.
The project team has already established a theoretical foundation for controlling the errors of downstream data analysis. They'll release their software tools by the end of March for the broader scientific community to use and evaluate.
The anticipation, Liu said, is that the outcome of this research can transform data reduction into something that is more trustworthy for science production, which is not the case today.
Update: April 1, 2022 - Liu recently won the National Science Foundation Career Award for his efforts.