Big Data in Business
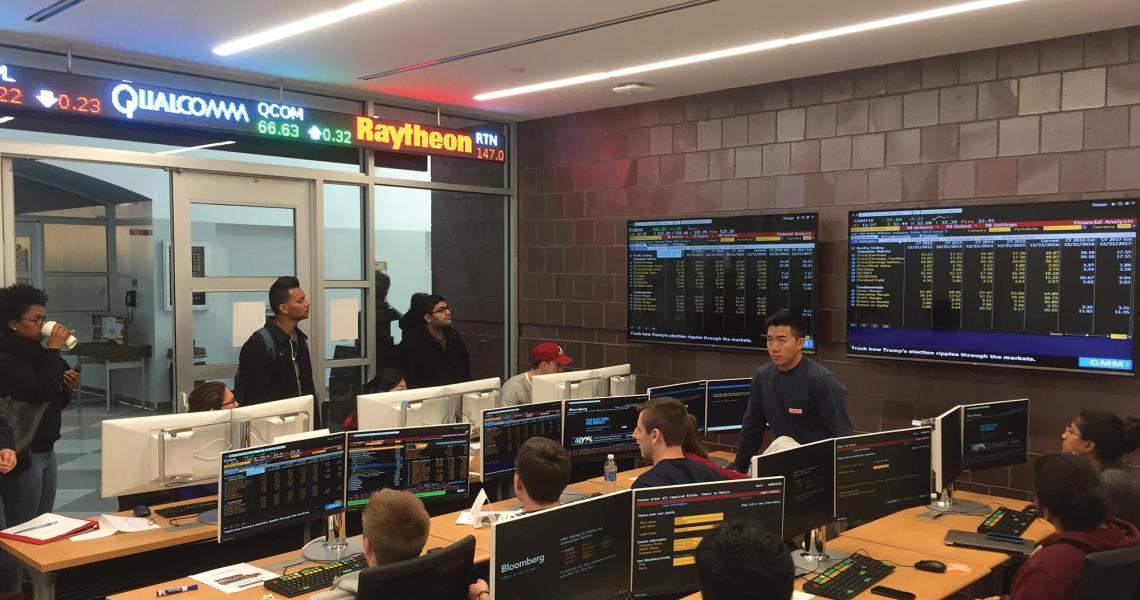
Today’s business executives, policymakers, researchers and consumers operate in an environment that is not only infused by information gleaned from big data, but increasingly directed by it. As decision makers turn to big data analytics tools to shape and support their initiatives, companies rely on it to hone and optimize their operations and shoppers to guide purchasing decisions.
The Martin Tuchman School of Management (MTSM) has aligned itself accordingly with several initiatives to support big-data research, something of a new direction for the school. In fall 2016, MTSM opened two new labs — the Ray Cassetta Financial Analysis Laboratory and the Business Analytics Laboratory — and launched a Ph.D. program in business data science that is one of the first in the country to integrate business analytics and management-systems theory with statistics, computing science and engineering.
To advance these programs, MTSM brought two new investigators into the fold: Associate Professors Dantong Yu and Maggie Cheng. Both are computer scientists with extensive experience in big data with applications in industry, scientific research and health care.
Cheng, who comes from Missouri University of Science and Technology, has been involved with two National Science Foundation-funded projects that use data analytics — one to develop algorithms to detect and diagnose in real time disruptions in electric power systems caused by outages and line-tripping, and the other to examine and predict the cyber behavior of individual users.
The algorithms she has developed for power systems “can also, with minor modification and extension, be applied to business,” says Cheng, noting that her contribution to MTSM’s big-data effort lies in “the integration of foundational research with the development of practical tools and software platforms” necessary to collaborate with colleagues who provide domain expertise for real-world business problems.
She is working with two Ph.D. students on methods for detecting fraud in the credit card and health insurance businesses, such as stolen cards and forged prescriptions. Changes that deviate from a customer’s habitual usage patterns can be detected with what is known as the change-point detection method, triggering further investigation. While some large organizations currently use anomaly-detection technology, there is still room for improvement on speed and accuracy. She is looking into effective real-time methods to alert the system as the first step, and down the road to develop means to distinguish between true frauds and false alerts.
Yu previously led the computer science group at Brookhaven National Laboratory’s (BNL) Computer Science Center, where he developed both novel data-mining algorithms that detect hidden interactions, patterns and anomalies in complex systems from the climate to genetics, as well as ultra-fast cloud-based data-transfer software. At NJIT, he is working on another BNL research award that deals with high-performance data sharing. In his research, he uses manifold-based spectral data analysis to detect anomalies and then taps his domain expertise to interpret them.
“I use deep-learning tools to discover patterns and classify digital images in the same way as domain experts,” says Yu, “I use this knowledge to guide and steer scientific experiments.”
For MTSM, he is studying time-sequence data related to the stock market and consumer sentiment. Temporal data is “powerful on the science side, but also on the business side,” Yu comments, noting that it can be used as a tool to predict financial bubbles and market volatility.
The key to their success will be accessing sufficient data. The Ray Cassetta lab will play an integral role in providing rich data, including temporal information about stock options information streaming in through the lab’s Bloomberg terminals.
Yu and Cheng both take an interdisciplinary approach to their research. He is on a team of physicists, experimentalists and computer scientists automating data processing and analysis with machine learning. She is the lead principla investigator on a team of mathematicians, engineers and computer scientists in her power-system research.
Learning about how other investigators view the world and gaining cross-disciplinary knowledge is vital to successful research, shares Yu. “Then you can actually solve a problem and define, understand and appreciate the results.”