NJIT Alum and 76ers Data Scientist on Joining the NBA's Data Revolution
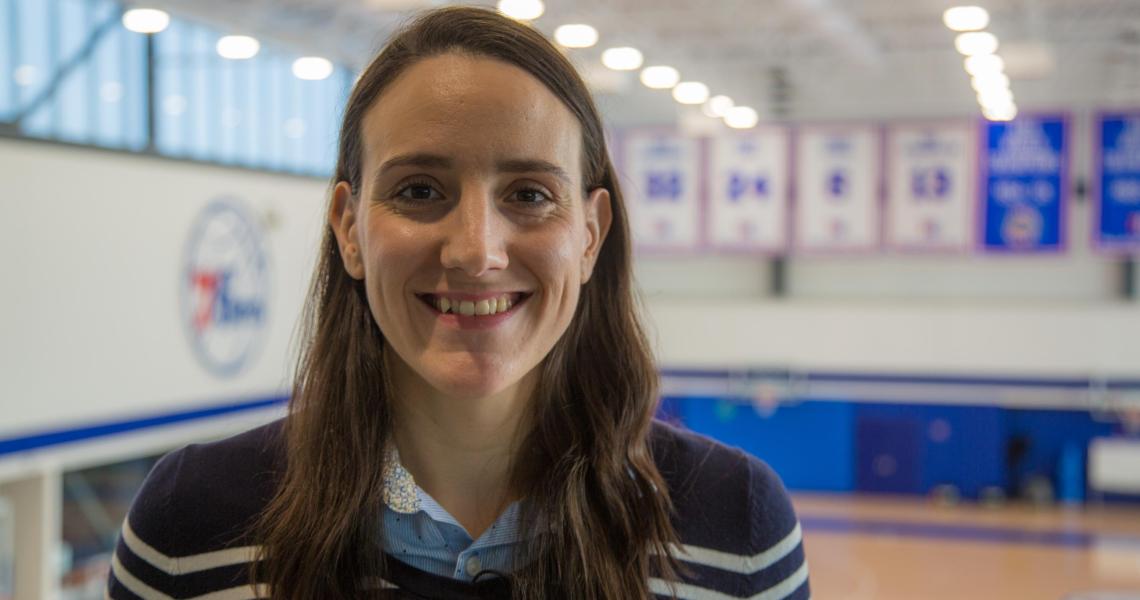
With the NBA’s 2020-21 season now underway, the Philadelphia 76ers stand as a perennial playoff team with title aspirations, unrecognizable from the team they were during three of the bleakest seasons the NBA has ever seen. A 47-199 record from 2013-2016.
What changed? Among the many changes, the organization hired a team of 10 mathematicians and computer scientists from around the world to assess, optimize, and even predict, each aspect of the team’s on-court performances through data — from smarter scouting of college players and undervalued free agents, to data-driven in-game play calling and pre-game strategies for how to line up on the court to exploit a particular opponent’s weaknesses.
Just months after graduating from NJIT in the spring of 2017 with a Ph.D. in computational fluid dynamics, Ivana Seric was among the stat experts hired by the 76ers, at the time joining one of the most expansive rosters of Ph.D. and MBA data scientists assembled by any team in the league.
We caught up with Seric to learn more about the science that goes on behind the scenes of the NBA’s bright lights and box scores.
Before joining the 76ers, you earned a Ph.D. in computational fluid dynamics at NJIT. What sparked that interest and what did your research entail?
Through a senior-year undergrad research project I became interested, particularly, in predicting or modeling fluid behavior on a solid surface — the type of research used for improving nano chips or solar panels.
During my Ph.D. research, I helped develop a novel numerical method for solving fluid equations including the Marangoni Effect, which occurs, for example, when liquids of different surface tension meet. You can see this when “tears of wine” streak down a wine glass … alcohol evaporates faster than water creating small pockets of liquid with higher concentration of water and with greater surface tension that forces fluid up the glass until droplets pool, become too heavy and fall back down. The model I helped build enabled us to quantify and visualize the dynamics of that effect.
I enjoyed working on these problems … coding, processing data, and creating models and visualizations that bring data to life.
What skills or experiences have you been able to apply from your academic background to be successful in the world of sports science?
My work at NJIT was very transferable to my role with the 76ers. I was coding and analyzing a lot data. Similarly, the modeling and simulation work both involve creativity and cross-processing. There is heavy analysis of the data but also the visualization of data…essentially taking all that data and making it into a cohesive story for others.
Being a basketball player for NJIT and the Croatia national team has also been very useful. I not only communicate with our analytics department, but I often speak with players and coaches in basketball terms to help break down what our data is telling us about our team’s performance or an upcoming opponent. It’s important they aren’t overloaded with information and can apply our insights during a game.
What does your day-to-day involve as a 76ers data scientist?
In the 76ers’ Research and Development Department, my role involves analyzing game data and attending coaches' meetings to report what trends we see in our team or an opponent. I prepare reports that involve data-based profiles of opponents, detailing their tendencies, what they struggle with and how we can exploit their weaknesses.
How have you seen data science transform the 76ers and the league generally?
Overall, the data that we have in the NBA today has really transformed how we are able to see game patterns and strategize. Until the 1990s, all we had was had box score data, which was purely outcome-based, such as points or rebounds. But the big shift recently is that we now have player-tracking data, which is action-driven. We can capture detailed interactions that lead to outcomes on the court. Having the data has given us much more possibilities on what we can learn about the game, what we can learn about our own team and our opponents.
How does your team use data to strategize?
In 2013, the NBA installed camera systems in every arena that track the X, Y coordinates of every player as they move around the court and X,Y, Z coordinates of the ball, including height of the ball in the air … all at a rate of 25 frames per second.
About an hour after each game ends, the league sends this data to every team. We get about a million rows of data a game. It’s enormous compared to the roughly 300 lines of box score and play-by-play data we were limited to before, and requires much more processing and machine learning skills. Along with player movement, we can tag interactions throughout games. For example, we can break down every pick-and-roll play in a game, the outcome, players involved, or defensive scheme deployed. We can collect and analyze these many types of data points for strategizing.
With the sports analytics market estimated to be worth more that $4 billion soon, what major technologies or trends do you see coming in the future?
Along with technology we have in terms of video processing and image recognition, a lot more may be possible beyond just tracking players’ X, Y location on the court. I can see advances in pose data, such as capturing players’ arm position, or to detect where a player is facing.
What do you enjoy most about your job now, and what would you like to do in the future?
I like to win! I truly enjoy working on basketball data and being involved in our on-court strategy. I want to do a lot more outreach events focused on girls who are interested in STEM and sports, but who may not think there is a future for them in pro sports or think they cannot make a living out of it. There is a big gap in the STEM fields. I just want to encourage young girls and women that finding success in these fields is doable.