Mathematical Epidemiologist Sara Del Valle '00 '01 Welcomed Back at 2020 CSLA Awards
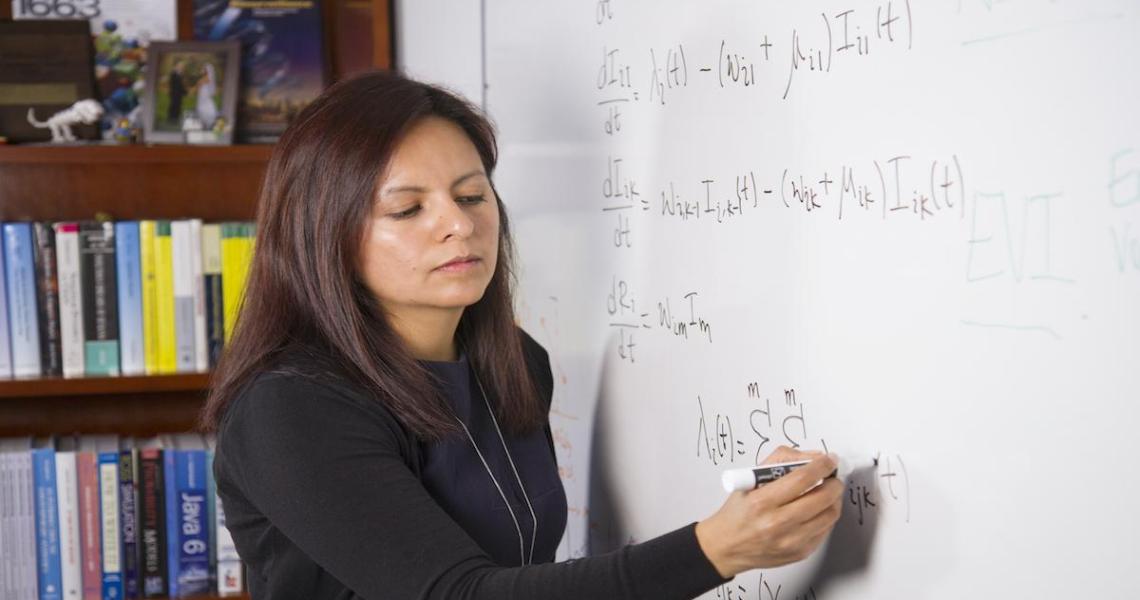
Sara Del Valle, a two-time NJIT alumna who works at Los Alamos National Laboratory (LANL) developing models for tracking and mitigating the spread of infectious diseases, gave the keynote address at the 2020 College of Science and Liberal Arts Awards ceremony.
CSLA Dean Kevin Belfield led the presentation at this year's ceremony, which continued its long tradition of awarding the outstanding achievements of students, faculty and staff across the college's diverse departments, from theater and history to physics and chemistry. The event is normally held in May but was instead held virtually on Nov. 11 due to the COVID-19 pandemic, making Del Valle a particularly appropriate choice as the event's special guest speaker.
We caught up with Del Valle, currently LANL's Deputy Group Leader in the Information Systems and Modeling Group, and asked her about her work as a mathematical and computational epidemiologist throughout the ongoing public health crisis, as well as her early career experiences at NJIT, where she received her bachelor's and master’s degrees in applied mathematics.
What inspired you to pursue a career in mathematical and computational epidemiology, and how did your time at NJIT help early on?
During my undergraduate studies at NJIT in the summer of 2000, I attended an REU (Research Experience for Undergraduate) program at Cornell University that focused on mathematical epidemiology. This program introduced me to how mathematics could be used to model and understand the spread of infectious diseases as well as to quantify the impact of mitigation strategies. The combination of math and epidemiology seemed really exciting to me because of the potential impact on public health. NJIT provided me with the tools, such as knowledge of differential equations and partial differential equations, that I now use to model the spread of epidemics.
What is involved in your role now at Los Alamos National Laboratory? And how have you been addressing the great challenges that have come with this current pandemic?
As a Deputy Group Leader for the Information Systems and Modeling Group at LANL, half of my time is spent overseeing over 40 scientists and 20 students and postdocs executing a diverse portfolio, from deterring proliferation of weapons-usable nuclear materials to infrastructure impacts and data analytics in support of national security. The other half is spent leading projects focused on developing mathematical, statistical and computational models for the spread of infectious diseases.
My team has been providing science-based decision support to state, local, and federal organizations such as the Center for Disease Control and Prevention, the Department of Energy, and the New Mexico Department of Health. We have been providing weekly COVID-19 forecasts and have been assessing the impact of non-pharmaceutical interventions such as masks and closures/reopening, as well as pharmaceutical such as testing and vaccines, on COVID-19 spread using various modeling approaches.
Could you explain more about how your team has used approaches from the weather forecasting community to better understand the spread of infectious disease, and what insights you’ve gained from this?
Forecasting is the ability to predict what will happen in the future based on analysis of the past and current data. The weather forecasting community leverages historical data and current observations from sensors around the globe (e.g., satellites, weather stations) and mathematical models to provide probabilistic forecasts of the weather, like hurricanes for instance. My team is borrowing approaches from the weather community and exploiting different types of data, including social media, climate, satellite imagery and disease surveillance data, and combining them with mathematical models to forecast infectious diseases.
Historically, mathematical epidemiology has focused on understanding disease spread and assessing the impact of “what if” scenarios. Disease forecasting is a new area that is still in its infancy when compared to weather forecasting, but it’s a growing field with great potential, especially since COVID-19 has put a spotlight on it. However, disease forecasting is challenging due the lack of surveillance systems on emergent behavioral response to epidemics. Individual decisions such as wearing a facemask can have an impact at the aggregate level, but it’s difficult to capture human behavior in real-time.
Therefore, there are limitations in terms of how far into the future we can forecast due to the uncertainty in human behavior, but our models appear to be robust in short-term forecasts. These forecasts are providing decision support that can help with resource allocation questions, targeted educational campaigns, and cost-benefit analysis of optimal intervention strategies.
Recently, you’ve also been using social media to study emergent human behavior on disease dynamics and in other disasters. How does your team use social media data, and what have you been able to learn through your analyses?
We have been using Internet data including social media to complement disease surveillance systems, identify emergent behavior and assess sentiment towards public health orders. We know people self-diagnose prior to going to the doctor, so we have been able to exploit Google search queries and Wikipedia access logs to assess the level of disease prevalence within a community.
Internet data is useful in providing real-time information due to reporting delays from official disease surveillance systems. Additionally, we have used Twitter data to identify emergent behavior, such as facemask usage, and sentiment towards vaccines. In the absence of official surveillance systems for human behavior in response to epidemics, we believe that Internet data streams can fill the gap, but they need to be complemented by disease surveillance and surveys, if available, to get a more representative sample of the population.
What technologies, modeling approaches, or other emerging solutions do think might be most impactful in mitigating disease spread looking ahead in your field, both for the current pandemic and new disease outbreaks in the future?
I think the combination of data-driven and model-driven approaches is what we need to tackle future threats and challenges. Though, a big challenge for this field is data integrity, so approaches that can tackle this problem will be useful to increase the accuracy of modeling results.
We are currently living in the data revolution era. But data can be misleading, as is evident from the epic failure of Google Flu Trends, because data cannot capture the mechanistic dynamics of how diseases spread. Mathematical models provide the foundation to understand how the world works but when combined with heterogeneous and dynamic data, the results are likely to be more accurate and robust. My team is developing hybrid models that combine machine learning approaches that exploit data with mechanistic mathematical models to build better models that can be flexible and can adapt to situations they weren’t anticipating. Just like the weather forecasting community, I think we need to combine different modeling approaches to see the whole picture and quantify uncertainty.