Computing Student Applied Neural Networks to Sound Waves as AMD Intern
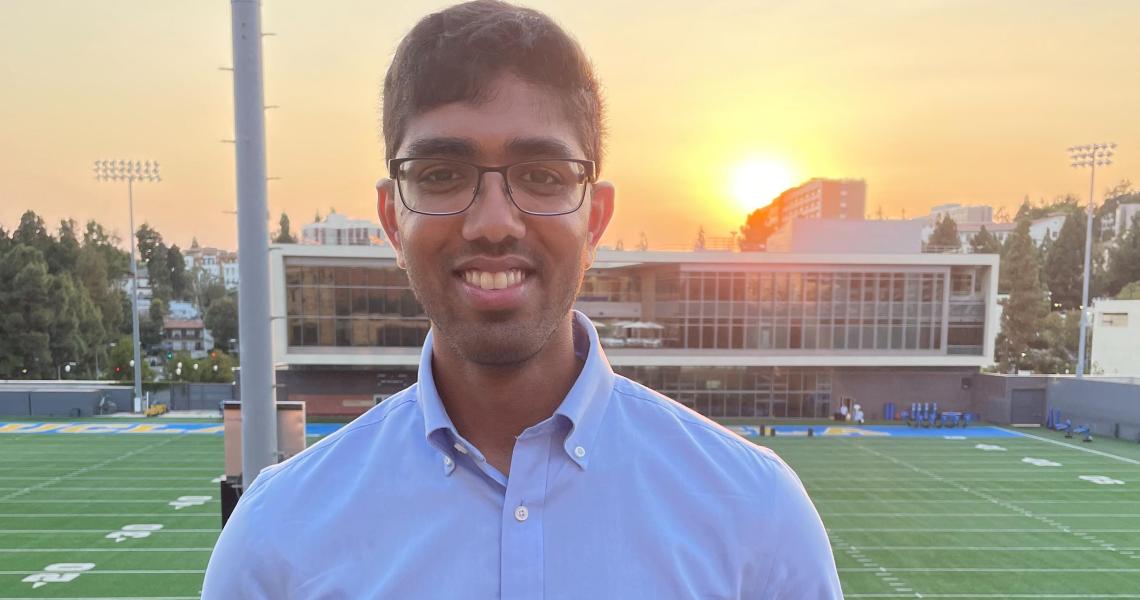
Bhargav Samineni loves applied math, and there are few better ways to see math in action than working on scientific computing problems with a mentor from a chip company like AMD.
Samineni interned last summer at the University of California/Los Angeles - Institute for Pure and Applied Mathematics, under guidance from an AMD scientific computing engineer in the application of neural networks to acoustic wave propagation, as an exercise in machine learning and a demonstration of the company's graphics processing units.
The NJIT senior from East Brunswick, an Albert Dorman Honors College scholar double majoring in computer science and applied mathematics, learned about this opportunity after deciding that research interested him more than programming. He approached Ying Wu College of Computing Professor Baruch Schieber, who is the computer science department chairman, to ask about the options for off-campus summer research. Then he applied to UCLA's Research in Industrial Projects for Students program which teamed him with four other students, from Johns Hopkins University, Louisiana State University, the University of Pennsylvania and UCLA.
AMD's Laurent White, computational scientist and principal software engineer, said he wanted to see the students engage in three phases during their brief internship period — study the basics of machine learning; address the problem of whether neural networks can evolve to out-pace traditional mathematical models of wave propagation; and if it works — analyze how and why.
Samineni intends to present the research results with his colleagues at an American Mathematical Society conference in Seattle this winter.
White said Samineni's team, none of which knew each other before the summer, worked much faster and more efficiently than expected. Some of their code led to better results than White's own code. They showed that a neural network can be trained to solve the wave equation. Much to White's surprise, they also made headway into the third phase by understanding how different parameter weights could be used as indicators for prediction quality, which is difficult to do even for industry professionals.
Samineni intends to present the research results with his colleagues at an American Mathematical Society conference in Seattle this winter. They're also presenting at the virtual NeurIPS conference. He'll graduate this coming spring, likely adding a machine learning course to his final semester while also applying to graduate schools for computer science.
Samineni suggested that if other NJIT students are interested in summer research experiences, they should get to know professors outside of class, start the application process as early as possible and apply to programs that are interesting even if they don't have relevant experience.
Less than 1% of students are accepted to the UCLA/IPAM program, White added, with Samineni blazing the trail for Highlanders.